One of the main problems of all drug discovery campaigns is the gigantic chemical space. The enormous number of the possible drug-like molecules makes it impossible to test all of them, let alone enumerate them. The increasing availability of in-vitro screening data and well-defined compound databases allows to search through certain parts of the chemical space, but huge parts remain inaccessible. One solution is de-novo drug design algorithms. Their main idea is to directly design new chemical compounds instead of screening through lists of already available ones. These algorithms require two main components. A scoring function to evaluate novel compounds and a rule to alter chemical structures. Depending on the available data, we use neural networks [1], QSAR models [2], and ligand docking [3] for scoring. The first two approaches require knowledge about already tested compounds for the same target, used as training data for activity prediction algorithms. If no preliminary screening data exists, we use ligand docking.
We can rely on our self-developed BioChemicalLibrary (BCL) for altering chemical compounds. It contains a powerful reaction and alchemy framework, providing an efficient infrastructure for handcrafting rulesets and defining reactions and reliable filters to remove unfavorable designs [4]. Combined, both scoring function and molecule altering allow for a very focused drug design approach to open up undefined chemical space and increase hit rates during in-vitro screenings.
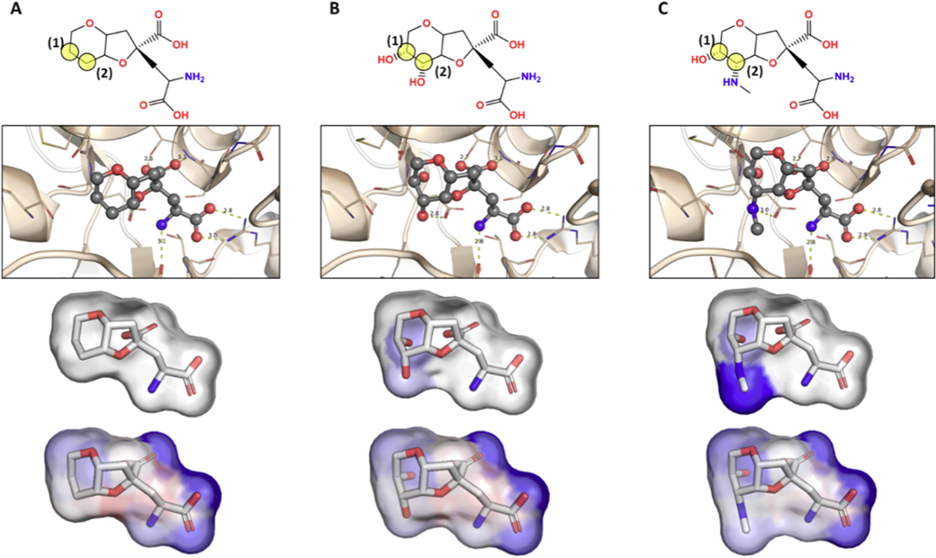
Figure 1: Pharmacophore maps of dysiherbaine analogues in complex with iGluR5 generated from BCL-AffinityNet. Labeled yellow transparent circles in the top panel are used to reference the substituted carbon atoms of interest. The second row illustrates each ligand in complex with iGluR5. The third row illustrates the common substructure pharmacophore map (i.e., pairwise per-substructure relative binding free energy changes). The fourth row illustrates the raw pharmacophore map for each ligand upon sequentially removing individual atoms and saturating open valences. [1]
References:
[1]
General Purpose Structure-Based Drug Discovery Neural Network Score Functions with Human-Interpretable Pharmacophore Maps, Benjamin P. Brown, Jeffrey Mendenhall, Alexander R. Geanes, and Jens Meiler, Journal of Chemical Information and Modeling 2021 61 (2), 603-620, DOI: 10.1021/acs.jcim.0c01001
[2]
Vu, O., Mendenhall, J., Altarawy, D. et al. BCL::Mol2D—a robust atom environment descriptor for QSAR modeling and lead optimization. J Comput Aided Mol Des33, 477–486 (2019). https://doi.org/10.1007/s10822-019-00199-8
[3]
Smith ST, Meiler J (2020) Assessing multiple score functions in Rosetta for drug discovery. PLoS ONE 15(10): e0240450. https://doi.org/10.1371/journal.pone.0240450
[4] to be published
[[ADD LINKS]]